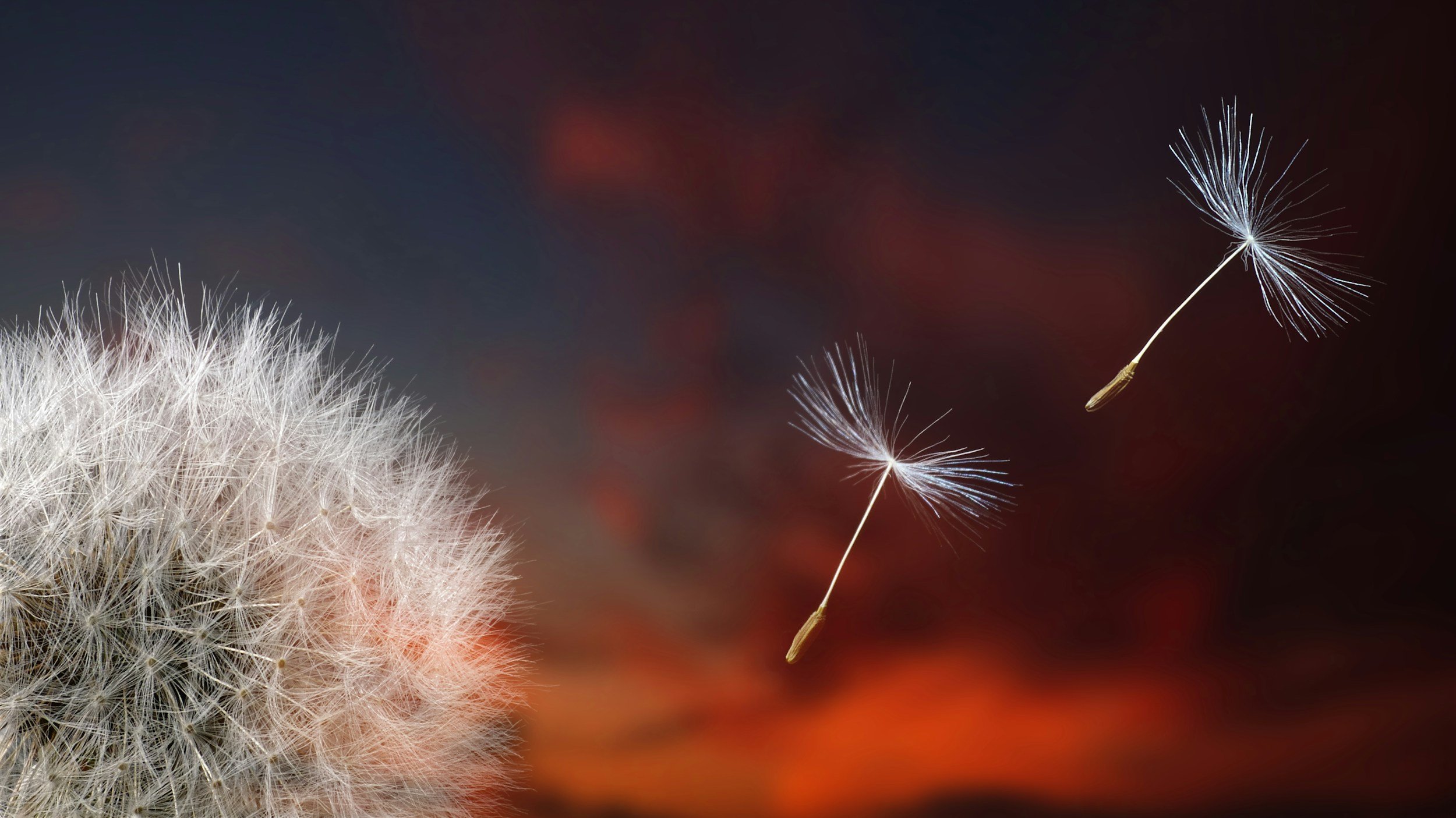
Dandelion Research
Measuring GLP-1 Efficacy in the Real World
Does real-world evidence show that GLP-1s are as effective as results reported in clinical trials? Which patient populations respond to GLP-1s outside of clinical trials, and which do not? We conducted one of the largest real world studies on GLP-1 usage and effectiveness. Read our latest research study below to learn more.
Pre-print currently under submission at medRxiv.
Or read the full research study below.
Real-World Efficacy of Glucagon-Like Peptide-1 Receptor Agonists for Weight Loss and Glycemic Control: A Retrospective Cohort Study
Neil Jethani, PhD^ and Shivaani Prakash, MSc, PhD^
^ Dandelion Health, Syosset, NY
Introduction
The high prevalence of both type 2 diabetes mellitus (T2DM) and obesity in the United States has wide-ranging implications for population health, clinical care, and health care costs. While a wide range of pharmacological treatments and disease management regimens exist to treat these conditions with varying degrees of efficacy, a promising new drug class known as glucagon-like peptide 1 receptor agonists (GLP-1s) and combination incretins such as dual GLP-1/glucose‐dependent insulinotropic polypeptides (GLP-1/GIPs, a subcategory of GLP-1s) has attracted widespread attention and interest from providers and patients for its potential to treat both these and adjacent conditions, and especially to drive weight loss. Both short- and long-acting GLP-1s have been shown to improve glycemic control and drive weight loss in a range of randomized controlled trials (RCTs). In recent years the US Food and Drug Administration has approved several different formulations of GLP-1s for treating patients with both conditions, among other adjacent indications.
While the results of randomized controlled trials are promising and indicate a significant positive impact for the management of both obesity and diabetes, there is limited real-world evidence on the efficacy of this key medication class in practice. There is especially a lack of evidence of whether GLP-1s are effective in the long-term for patients in comparison to real-world controls who are managing their conditions with other interventions over time that may also result in weight loss or better glycemic control. The magnitude of weight loss in particular, as observed in clinical trials and as popularized across social media, may vary in the real-world and among patients with different backgrounds, clinical profiles and history as compared to protocolized weight loss clinical trials. In part, the paucity of evidence on this topic is related to the lack of rich clinical data on a broad range of patients with enough long-term data following use of these medications for indications such as obesity.
In this study, we used causal inference methods to conduct an analysis of real-world long-term efficacy of GLP-1s, with a retrospective comparison of those continuously prescribed GLP-1s to a matched set of real-world controls with similar baseline characteristics who did not manage their conditions with GLP-1s. The study compared the real-world outcomes of GLP-1 patients to the real-world outcomes of patients who were similarly likely to initiate use of a GLP-1, but did not. We compared primary outcomes that were analogous to those reported in clinical trials; namely, changes in percent hemoglobin A1c (HbA1c) levels to assess glycemic control, as well as percent reduction in weight to assess weight loss, in order to allow for comparability of measures.
Methods
Data
This study utilized a subset of Dandelion Data, a database of regularly updated clinical data from 2016 to the present from a consortium of US non-academic healthcare systems. The database includes patient-level electronic health record (EHR) data on demographics, diagnoses, procedures, health system encounters, medication orders and administration, laboratory and diagnostic test orders and results (e.g., glucose and potassium tests and values) and vital signs (e.g., heart rate and blood pressure). These data are linked to unstructured data such as medical imaging (e.g., CTs, MRIs, XRays, Ultrasounds), associated radiology reports, ECG waveforms, echocardiograms and clinical notes, to create a longitudinal, multimodal dataset capturing the trajectory of clinical care. Prior to extraction from a health care system, Dandelion data are de-identified via privacy-preserving methodologies that are specifically developed for each data type and approved by expert determination under the HIPAA Privacy Rule.
Once extracted, data are harmonized across healthcare systems into a unique data model through semantic processing and a data transformation layer, and then made available for analysis on the Dandelion platform. The data utilized for this study were primarily drawn from EHRs accessed in January 2024. The data included observations from January 2019 - October 2023 to ensure a contemporary dataset reflective of current clinical practices and recent GLP-1 uptake.
Study Design and Participants
Patients were identified based on inclusion criteria to generate two cohorts to assess the comparative effects of GLP-1 therapies as compared to non-GLP-1 real-world care for this retrospective cohort study. The approach to participant selection underpinned the study's objective to delineate the specific contributions of GLP-1 therapies to clinical outcomes within a real-world context, ensuring comparability and minimizing confounding across cohorts.
The GLP-1 (“treatment”) cohort was comprised of individuals who (i) had no prior history of GLP-1 use, (ii) initiated GLP-1 medications from January 2019 onwards with the prescription originating from a primary care provider or endocrinologist, and (iii) had medication orders indicating a minimum duration of 12 months of prescriptions. In addition, treatment cohort patients were required to have a minimum of one outpatient visit prior to initiation, and one follow-up visit within six to twelve months following GLP-1 initiation, ensuring a comprehensive evaluation of the therapy's sustained impact in an uncensored population. The first GLP-1 medication order was considered the treatment initiation and the date of that medication order was designated as the patient’s index date.
The real-world “control” cohort consisted of individuals with no GLP-1 medication history who still engaged with the healthcare system to a comparable extent, as marked by outpatient encounters and a history of medication use. Control cohort patients had at least one outpatient encounter with a primary care provider or endocrinologist during the study period, a history of at least one non-GLP-1 medication prescription, and a follow-up within six to twelve months from a randomly selected index date to mirror the treatment cohort's follow-up schedule.
Patients were followed to assess primary and secondary study outcomes (described in detail in Measures below) in aggregate at 3, 6, 9 and 12 months after index date.
Measures
Exposure
Exposure was defined based on GLP-1 medication usage, as determined by medication order records for prescriptions and refills in the EHR. Patients included in our treatment cohort were required to have a minimum of 12 months of continuous duration of GLP-1 prescriptions in order to limit the treatment sample to those who were most likely to continue care. This allowed for assessment of the impact of long-term use, and contextualized use as compared to clinical trials. To reflect real-world prescribing patterns, no specific medication administration protocol was mandated for inclusion given that different dosing and titration schedules are utilized for GLP-1s in real-world clinical practice. Patients in the control cohort had no GLP-1 prescription history. This enabled evaluation of GLP-1's impact relative to standard care, which may include other anti-diabetic or weight loss interventions.
Outcomes
Primary outcome measures were selected based on available vital and lab measurements that would capture the intended impact of GLP-1s especially for diabetic or obese patients, as at the time of this study, GLP-1 use had primarily been approved as therapy to improve glycemic control and support weight loss. Specifically, the primary outcome measures of interest were HbA1c (A1c) levels and weight in pounds assessed over time, both in absolute terms and as a percent change. The most recent measurements taken prior to the index date were documented as the baseline values for HbA1c and weight.
Patient Demographics and Clinical Variables
Patient demographics and clinical characteristics were assessed at or prior to the index date, to establish baseline profiles for each participant. Demographic variables included age, sex, race/ethnicity, and marital status. Clinical characteristics from EHR data such as relevant comorbid conditions, medication history and prior procedures were included if captured within the two years prior to the index date. This helped to understand the broader health context of the study population and enable selection of a true “control” population.
These baseline characteristics and comorbidity profiles provided a comprehensive overview of the study population, allowing for nuanced analysis of GLP-1 medication effects to account for the differences in a diverse patient cohort with varying health backgrounds.
Statistical Analysis
Propensity-Score Matching
To control for potential confounding and ensure comparability between the treatment and control groups, a 1:1 Propensity-Score Matching (PSM) approach was implemented to select patients in the control cohort who were most similar to those in the treatment cohort. First, multiple imputation modeling was employed to address missing baseline covariates. This enhanced the robustness of the matching process. Matching was based on a propensity-score model that utilized 42 observable baseline covariates, and matched controls were selected based on a caliper width of 0.1. The propensity scores were modeled using a machine learning method called eXtreme Gradient Boosting (XGBoost), which uses a decision tree ensemble approach to optimize the balance of baseline covariates utilized for matching. We applied XGBoost with 5-fold cross-validation to ensure good generalization and calibration across test and validation sets. The acceptable standard mean difference (SMD) threshold for covariate-level comparisons between treatment and control cohorts was set at 0.1 to ensure balance between treatment and control cohorts.
Treatment Effect Estimation
The average treatment effect of GLP-1 medications on the primary and secondary outcomes was evaluated using regression models that estimated the outcomes at 3, 6, 9 and 12-months after GLP-1 initiation in the treatment cohort, as compared to the same time-points after index date for the matched controls. We evaluated Estimated Treatment Difference (ETD) for each outcome to compare results between treatment and control groups and assessed this difference for statistical significance.
To assess glycemic control over time, HbA1c was modeled as a continuous outcome (i.e., absolute HbA1c value at each time point, absolute change in HbA1c value at each time point relative to index date measurement) and a binary outcome (i.e., percent of cohort with HbA1c < 7% at each time point, which is a commonly used proxy clinical signal for achieving good glycemic control).
Weight loss was also modeled as a continuous outcome (i.e., percent weight loss at each time point relative to index date measurement) and a binary outcome (i.e., percent of cohort achieving 5%, 10% and 15% weight loss at each time point relative to index date measurement).
Continuous outcomes were analyzed using linear regression models, whereas binary outcomes were assessed with logistic regression models. To account for missing outcome data at different follow-up time points, Inverse Probability Weighting (IPW) models were utilized based on the extended set of 42 baseline covariates and available outcomes as predictors. This comprehensively handled missing outcomes data and established trends for patients over time.
Subgroup Analysis
In addition to aggregate treatment effect estimation between the treatment and control group, subgroup analyses were conducted to explore the differential effects of GLP-1 treatment on primary outcomes across various patient segments. For each subgroup analysis, propensity-score matching with machine learning methods was repeated specifically within the subgroups of interest to construct treatment and control cohorts. Then treatment effects were re-estimated for the subgroups.
Given that GLP-1s are approved for patients with type 2 diabetes and obesity, and the treatment goals for these two groups may differ, we assessed the primary outcomes separately by indication. A subgroup analysis was conducted for those with T2DM only, those with obesity or overweight only, and those with both indications, based on diagnosis at or prior to index date. Very few patients in the cohort had neither diagnosis. These patients were excluded from the subgroup analysis.
Further stratification for matching and treatment effect estimation was done across key demographic factors such as age, sex, and race/ethnicity to evaluate the consistency of treatment efficacy across diverse patient subgroups.
Finally, the treatment cohort was divided into decile subgroups by magnitude of change in primary outcomes, to evaluate and compare the range of responses to GLP-1s over time.
Sensitivity Analysis
Several sensitivity analyses were performed to confirm the robustness of our findings. First, to ensure that the matching methodology was not a key driver of estimated treatment effects, we compared the results for modeling our primary outcomes for matched treatment and control groups selected through Coarsened Exact Matching (CEM), in addition to Propensity Score Matching.
To ensure that our results were robust to the imputation methodology utilized for missing data, we tested additional data imputation methods beyond multiple imputation, including Random Forest (RF), K Nearest Neighbor (KNN), and Bayesian Ridge Regression (BR) estimation. Findings from modeling the efficacy of GLP-1s on the primary outcomes for the overall treatment and control cohorts were compared after using these methods to ensure that they were directionally consistent.
Results
Baseline Cohort Characteristics
In total, 3040 patients met the inclusion criteria for the treatment cohort, and these were matched 1:1 to 3040 corresponding patients to create the control cohort. Demographic characteristics for the matched cohorts are shown in Table 1.
Across both cohorts, the median age was 57 years, and a slightly larger proportion were female (54.4%). The cohorts were predominantly Hispanic (32.9%) and White, non-Hispanic (31.6%) patients. No significant imbalance was detected across racial/ethnic groups between treatment and control patients. Comorbidities and medication use history showed small, statistically insignificant differences between groups (i.e., SMD ranging from 0.001 to 0.047 and from 0.007 to 0.031, respectively), substantiating the quality of the cohort matching. Pre-index HbA1c and BMI were also balanced between the cohorts post-matching (i.e SMD of -0.021 and 0.055, respectively), suggesting that the PSM process had effectively identified control patients who had similar profiles as GLP-1 patients at index date for the study.
Table 1. Baseline Characteristics and Covariate Balance of the GLP-1 Treatment Cohort and Matched Control Cohort
Primary Outcomes: Glycemic Control and Weight Management
Weight loss was observed in both the treatment and control cohorts over time, but the magnitude of weight loss was more substantial in the treatment group at all measured timepoints with a statistically significant ETD of -1.42% (95% CI: -2.10, -0.73; p < 0.001) between the groups at 12 months post-index date (Table 2).
Table 2. Impact of GLP-1 Medications on Weight Loss and Glycemic Controls Compared to Matched Controls over a 12-month Period
*** indicates p < 0.001; ** indicates p < 0.01, * indicates p < 0.5
A greater number of patients in the treatment cohort also reached the weight loss thresholds of 5%, 10%, and 20% weight reduction from baseline weight throughout the 12-month period of the study as compared to the control group (Figure 1), with a measurably larger prevalence of weight loss in the treatment group evident at each follow-up time point.
Figure 1. Percentage of Patients Achieving Various Weight Loss Thresholds (5%, 10%, 20%) Over Time among Treatment Cohort Compared to Matched Controls
Overall, those taking GLP-1 medications also saw an incremental decrease in HbA1c levels at each time point over the 12-month period as compared to those who were not taking GLP-1 medications (Table 2), with statistically significant differences in HbA1C between groups observed at 3, 6 and 9 months after index date. The proportion of patients achieving an HbA1C below 7% was also higher in the treatment group as compared to the control group at every time point (Figure 2). The greatest difference in glycemic control between groups was observed in the first 3-6 months of GLP-1 use, but the difference in the proportion of patients achieving HbA1C < 7% between these two groups continued to increase over time, suggesting sustained and improved glycemic control among patients taking GLP-1s.
Figure 2. Percentage of Patients Achieving HbA1C below 7% Over Time among Treatment Cohort Compared to Matched Controls
Weight Loss and Glycemic Control Across Patient Subgroups
Table 3 and Figures 3a-3d present the subgroup-specific weight loss outcomes over 12 months. While both overweight and T2DM patients taking GLP-1s experienced significant weight loss as compared to matched controls (p<0.05 for all subgroups), the overall magnitude of ETD was greatest for those taking GLP-1s with a diagnosis of obesity alone, as compared to those diagnosed with T2DM only or both T2DM and obesity.
Table 3. Impact of GLP-1 Medications on Weight Loss Across Different Subgroups Relative to Matched Controls, Assessed by Mean Percent Change in Weight After 12 Months and Estimated Treatment Difference (ETD).
*** indicates p < 0.001; ** indicates p < 0.01, * indicates p < 0.5
Figure 3. Impact of GLP-1 Medications on Weight Loss Across Different Subgroups Relative to Matched Controls, Assessed by Mean Percent Change in Weight After 12 Months: (a) Patient Age Group; (b) Patient Diagnosis—Type 2 Diabetes Mellitus (T2DM), Overweight/Obesity, or Both; (c) Patient Race/Ethnicity; (d) Patient Sex.
A greater ETD in terms of weight loss over 12 months was observed in females as compared to males (-1.63% vs. -1.02%), although the ETD was statistically significant for both groups (p<0.01). An age-related trend in ETD was noted, with statistically insignificant ETDs amongst those aged 18-30 and 30-40, but significant differences amongst age groups above 40 years old. The most pronounced effect was observed in the 70+ age subgroup (ETD = -2.94%, p < 0.001). Slight differences in weight loss between treatment and control patients also emerged within racial/ethnic subgroups, with the Hispanic and Other/Unknown subgroups experiencing weight reductions with ETDs of -1.49% (p < 0.002) and -2.92% (p < 0.004), respectively. Magnitude of weight loss at 12 months was relatively consistent across racial/ethnic subgroups.
Analysis of GLP-1 medication effects on HbA1c levels across demographic subgroups showed consistent efficacy over 12 months (Table 4). Notably, patients with both T2DM and overweight/obesity conditions experienced a significant ETD in HbA1c reduction (ETD = -0.14, p = 0.016), contrasted with the non-significant change in patients with T2DM only (ETD = 0.08, p = 0.719). This suggests that GLP-1 therapies are particularly effective in managing glycemic control in patients with comorbid obesity or overweight.
Table 4. Impact of GLP-1 Medications on Glycemic Control in Different Subgroups Relative to Matched Controls, Assessed by Mean Change in HbA1c After 12 Months and Estimated Treatment Difference (ETD)
* indicates p < 0.05
A final subgroup analysis (Figure 4) looked at subgroups based on the degree of response by classifying the treatment cohort into deciles based on their magnitude of change for the primary outcomes of percent change in weight and HbA1C over time, and then assessing the trend in outcomes over time for the top 10% and bottom 10% of responders. For percent weight loss, we see that the top 10% of the treatment cohort had lost up to 15% of their weight by 12 months, as compared to almost 5% average weight gain in the bottom 10% of respondents. Similarly, for percent HbA1c, we see a dramatic reduction from average HbA1C at baseline (10.5%) to well-managed glycemic control (HbA1C <7%) for the top 10% of respondents by 12 months, whereas the bottom 10% of respondents maintained or slightly increased HbA1C in the 12-month period and on average, with average HbA1c levels around 8 - 8.5% observed.
Figure 4. Percent HbA1C and Percent Change in Weight over 12 Months Among Top 10% and Bottom 10% Responders in GLP-1 Treatment Cohort Only
Sensitivity Analysis
Sensitivity analysis testing different matching methods yielded similar overall results for ETD among primary outcomes, both in terms of magnitude and statistical significance, suggesting that our findings were not sensitive to the use of PSM as compared to Coarsened Exact Matching. Similarly, the key findings of the study were robust to a range of data imputation methods tested, with no drastic changes in direction or ETD magnitude observed (results available upon request). These analytical strategies allowed for a thorough examination of the effects of GLP-1 medications on key outcomes, while ensuring the robustness and reliability of the findings through comprehensive sensitivity analyses.
Discussion
In this study, we conducted a real-world assessment of GLP-1 efficacy over 12 months among US adults as compared to a matched set of controls who were clinically similar but likely pursued other therapeutic options to manage their weight and HbA1c.
We find that at every time point assessed in the study (3, 6, 9 and 12 months) following GLP-1 initiation, the GLP-1 treatment cohort achieved a greater magnitude of total weight loss and HbA1C reduction, and a greater proportion of those taking GLP-1s achieved outcomes such as 5%, 10% and 15% weight loss at each follow-up point, as compared to controls. Significant variation was seen in the magnitude of weight loss and glycemic control both (i) between treatment and control cohorts when stratified by indication, age, sex and race/ethnicity, and (ii) within the GLP-1 treatment cohort itself, as shown by the stark differences between the top and bottom 10% of responders. This suggests that there are significant variations in the rate of response over time amongst GLP-1 patients. To our knowledge, this study presents the first real-world efficacy study of any modern GLP-1 use as compared to regular care management in a control group, facilitated by the availability of recent outcomes data up to 2024.
Findings in our study are directionally consistent with Randomized Controlled Trials (RCT) that assess differences between GLP-1 patients and a control group over time, such as the STEP and SUSTAIN series of trials. However, our study also reveals nuanced differences when compared to outcomes reported in RCTs. Notably, treatment cohorts in RCTs typically report more pronounced effects than controls for the primary outcomes we assessed, and in our real-world study, there was a clear continuum of responsiveness to GLP-1s, suggesting a high degree of variation in terms of patient benefit from GLP-1s.
In the current study, the treatment group demonstrated a statistically significant degree of average weight loss (3.1%) at 12 months, with subgroup analyses revealing varying degrees of weight reduction across demographic groups. The most pronounced weight loss was seen in the age 70+ subgroup (3.61%; ETD of -2.94%), but the overall magnitude of weight loss was still less than what was reported in the STEP and SUSTAIN trials, where average weight reductions reached ranges of 5-15% (ETD of -2.6% to -12.5%) over a similar duration of treatment with high-dose GLP-1s.
Similar trends were observed for glycemic control outcomes. This study found a modest but statistically significant reduction in HbA1c levels overall (i.e., average reduction of 1.1% with a -0.11% ETD at 12 months). This is slightly lower than the reductions reported in RCTs, where HbA1c decreases ranged from -0.5% to -1.8% (ETD of -0.3% to -1.5%), depending on the trial and semaglutide dosage (e.g., STEP, SUSTAIN 5). The results in our study that were most analogous to RCT results were among the top 10% of treated respondents for weight loss (i.e., average reduction of 15% at 12 months) and HbA1c (i.e., average reduction of 3% at 12 months), while the bottom 10% of treated respondents saw no change or slightly worsening outcomes over time.
There are a number of reasons why real-world use and efficacy may differ from observed impacts in RCTs such as the STEP and SUSTAIN trials. First, in this study, we did not restrict either the GLP-1 treatment cohort or the control cohort to any specific medication regimen, dosage trajectory or adjunctive interventions, as the intent was to capture the full range of possible real-world medication usage that is occurring, especially as uptake increases in the US population. There is evidence that titrating GLP-1 dosage and switching between GLP-1 medications can occur in clinical practice due to factors such as lack of desired impact, patient preference, insurance coverage and side effects. There is also evidence of the opposing phenomenon, therapeutic inertia, where there is a delay in intensifying treatment despite the availability of effective options. The degree to which any of these factors impacted weight loss or glycemic control outcomes was not assessed in this study, but it is likely that variation in the therapeutic regimens pursued by the treatment group in order to reach desired outcomes impacted their trajectory, and could diminish the real-world effectiveness of these therapies.
Second, RCT patient populations are composed of carefully selected patients who meet stringent inclusion and exclusion criteria that are specific to the indication and medication being evaluated, such that they are the best candidates to test a new therapy. This is by design, and reflects the necessity of RCTs to assess clinical impact for a specific therapeutic and indication. This often means that the control arm in RCTs is also subject to stricter, medication-based therapy. The difference between the population recruited for an RCT as compared to our study population impacts our findings in two clear ways. The treatment cohort in our study is not subject to the same stringent criteria when taking medications as those in a trial. This is especially consequential for GLP-1s, where a significant increase in public interest in this medication class for weight loss has been accompanied by a sharp rise in initiation by patients from different backgrounds. From an analysis of Dandelion data, the number of new GLP-1 patients has increased by 7-10% monthly over the course of 2023-2024. This wider range of treated patients is likely to see attenuated effects of GLP-1s as compared to an analogous treatment cohort in an RCT. Among the control cohort, these patients are likely pursuing other interventions (e.g., lifestyle, diet, exercise) in addition to medications aside from GLP-1s that could lead them to see beneficial results. The intent with propensity-score matching was to identify control patients that were just as likely to have been prescribed GLP-1s as the treatment cohort based on a wide range of observed covariates, and it is reasonable to assume that such patients would be pursuing additional therapies over the study duration that would improve their weight and HbA1c as well. Both of these effects likely reduce the ETD in a real-world context as compared to an RCT.
Third, differences might be explained by adherence rates and monitoring, as the stricter monitoring and controlled environments in RCTs - which enhance medication efficacy and patient compliance - are generally not replicable in real-world settings.The high variability in adherence and patient management in real-world scenarios likely dilutes the potential maximum efficacy seen under controlled trial conditions. In a study of real-world use of GLP-1s, Weiss et al. (2022) and Palanca et al (2023) reported that despite the potential for improved glycemic control with GLP-1 receptor agonists, persistence and suboptimal adherence were significant issues, resulting in a marked decline in treatment persistence over a two-year timeframe. The role of adherence and persistence is beyond the scope of this study but a promising area of further research is to explore which patients may benefit from this medication class, and how to ensure that the benefits of GLP-1 medications are fully realized in a real-world setting by addressing poor adherence.
Finally, we saw clear variation in ETD by subgroups, including by demographic and clinical features. Observed differences in weight loss were significant for those who had a diagnosis of obesity (either or without comorbid T2DM), but not for those with T2DM only. Conversely, differences in glycemic control were significant only for those who were diagnosed with both obesity and T2DM, but not for those with either obesity alone or T2DM alone. This suggests that the indication and reason for taking GLP-1s likely play a role in effectiveness, as those focused on only glycemic control may not see any change in weight loss, and vice versa. The treatment differences by age, sex and race/ethnicity that we found suggest that patient profiles should be evaluated before pursuing treatment with GLP-1s. For example, we saw significant ETD among patients aged 70+; however, this is also a patient population that is at risk for fractures, which can be a side effect of some GLP-1s. Studies of heterogeneous effects such as ours can further enable a precision medicine approach to clinical care for obesity and diabetes, to ensure that the right patients are receiving the right therapeutics based on their condition.
The variability found in our results is also consistent with RCT results demonstrating a continuum of effects - while overall ETD for weight loss was attenuated in our study, the RCTs for semaglutide and tirzepatide have also shown that between 9-14% of patients lost less than 5% of body weight over 12 months, and between 16-33% lost less than 10%. Our findings are also consistent overall with the few published studies on real-world GLP-1 use; for example, White et al. (2023) found that while significant weight loss was achievable with GLP-1s among type 2 diabetes patients, the average weight reduction was relatively modest (2.2% over 72 weeks). This highlights the variability in patient response in less controlled real-world environments. Recently, others have reported on this variation in practice, with some physicians estimating that 10-15% of GLP-1 patients are “non-responders” in their patient population.
Strengths and Limitations
This study has numerous strengths to highlight. Our analysis included a matched treatment and control cohort that was followed longitudinally for a minimum of 12 months, with a rich range of covariates from source-of-truth clinical data to match patients with a machine learning approach that allowed for strong overlap between the treatment and control cohorts. This allowed for inclusion of a broad range of GLP-1 patients and strong real-world outcomes assessment with well-powered subgroup analyses by key patient features and indications.
The Dandelion dataset also allows for up-to-date analyses that do not suffer from the same delays as more traditional survey-based or claims-based analyses, allowing us to focus on the long-term efficacy of approved GLP-1s through the end of 2023 for this study. Further, our treatment effects were directionally consistent when differing matching and imputation methods were used before modeling treatment effect, suggesting that our findings are robust to the specification used.
There are several limitations to note in this study. The largest of these limitations is the lack of external pharmacy dispense data, in order to verify that medication orders had been filled over the course of the study, which could more closely signal adherence. While the Dandelion algorithm is able to combine measures from medication orders to assess the estimated duration of treatment as prescribed by a physician, we cannot speak to the downstream behavior at the patient level. In order to address this limitation, we implemented a fairly strict definition of GLP-1 use to include patients in the treatment cohort: medication orders indicated 12 continuous months of prescribed GLP-1 use. Given that the use of modern GLP-1s is fairly new, we believe that those with 12 continuous months of orders and follow-up outpatient encounters in our data would not yet be receiving these prescriptions without a clear need for this therapy and with supervision or follow-up care from a physician. As such, this is likely capturing true users. Since it is difficult to confirm adherence without accompanying dispense data for these patients, we anticipate these data to become a part of the Dandelion dataset in the near future.
Another limitation to note is the missing outcomes data at all follow-up time points for all patients. While an RCT has consistent monitoring and more complete follow-up data, we had more sparse outcomes data that we leveraged to assess the trends between the treatment and control cohorts at each time point. Our findings were robust to the imputation methodology utilized to address this, and we did not find evidence that missingness was associated with any particular patient type or profile, but to the extent that there may be a pattern to the missingness in the availability of covariates or outcomes data, this may impact the magnitude of our findings.
Similarly, residual confounding may exist; even though the treatment and control cohorts were drawn from the same patient population in the same geographic area during roughly the same time period. Unobserved differences in motivation for initiating GLP-1s, cost of medications given differences in insurance coverage, and other lifestyle factors might play a role. Given the robustness of our findings, the residual confounding would need to be quite strong to nullify the findings, especially for weight loss.
Finally, while the primary outcomes we selected (weight loss and HbA1c) were consistent with clinical trial endpoints, they are limited in their ability to analyze early and true physiological impacts of GLP-1s such as changes to visceral and subcutaneous fat. For both of these final limitations, the use of rich multimodal clinical data such as clinical notes to assess patient-level features driving motivation for GLP-1 use and discontinuation, as well as imaging to quantify and assess fat loss through CT scans could help shed light on the true impact of GLP-1s on body composition as well as patient profiles that are related to varying levels of treatment effect.
Future Research
Given our findings, future research should focus on investigating the heterogeneity in treatment response to GLP-1s, modeling predictors of responsiveness, and analyzing unstructured clinical data to understand the impact of GLP-1s on measures beyond weight loss and HbA1c (e.g., loss or preservation of lean body mass). This research could support the development of personalized medicine approaches and shed light on the value of continued monitoring and adjustment of treatment protocols based on individual patient needs and responses. In addition, more work is needed to identify the interaction between short-term and long-term use and outcomes. While our study inherently focused on long-term use, both for comparability to RCT results and also to reduce the limitation imposed by the lack of dispense data, additional analyses with Dandelion data linked to external pharmacy dispense data could enable more specific analysis of drivers of adherence and persistence with GLP-1 therapies, and whether the heterogeneity of effects are still observed in the short-term. Finally, there is a need to leverage multimodal clinical data beyond structured EHR findings to truly understand the holistic impact of GLP-1s with outcomes captured from different types of structured and unstructured data. This approach not only helps in aligning clinical expectations but also supports the optimization of treatment strategies to achieve the best possible outcomes for patients. As this field evolves, ongoing research and dialogue among healthcare providers, patients, payers, and regulators are necessary to ensure that diabetes and obesity management are effective for diverse patient subgroups.
Conclusion
In this real-world efficacy study, we find significant estimated treatment differences in weight loss and HbA1c reduction between patients taking GLP-1s for 12 months as compared to propensity-score matched controls followed for a similar period of time. Further, we find a continuum of responsiveness among GLP-1 patients and significant variation by patient subgroup. The differences observed can be attributed to several factors inherent to real-world studies as compared to RCTs, and future work is needed to further characterize patient profiles most likely to benefit from GLP-1s with findings from both structured and unstructured clinical data modalities. The integration of findings from both RCTs and real-world studies is essential for a holistic understanding of GLP-1 medication effects and for development of comprehensive strategies to support patients and healthcare providers in managing obesity and type 2 diabetes, especially as utilization of this medication class continues to increase over time.
Acknowledgements
The authors would like to acknowledge the invaluable support and contributions of Kiko Wemmer, Man Qing Liang and Jamie Dermon, MD in developing this study and manuscript.